Industry leading credit risk models
Valuatum SaaS credit risk analysis software revolutionizes credit rating process through advanced automation and dynamic credit risk models. Valuatum offers the most accurate credit ratings including automated analysis and reporting. Valuatum’s platform creates value through the credit value chain from credit applicant to investors. You can access our credit risk presentation here.
SPEED UP CREDIT APPLICATION PROCESS
Receive pre-rated credit applications and reports for further analysis
Improve the average quality of credit applications
Generate different types of automatic reports
STATE-OF-THE ART BANKRUPTCY MODELS
Based on advanced machine-learning algorithms
Models adapt to situations where traditional models fail
No more missed opportunities or decisions based on misleading information
VERSATILE CREDIT ANALYSIS PLATFORM
Access and edit your data anywhere via web browser, Excel models, or APIs
Platform can be tailored to fit your needs exactly
Access to audited financial data of nearly 200 000 Finnish companies
Valuatum’s platform creates value to all participants of the credit supply chain

Easily sent your credit application to multiple lenders through Valuatum’s platform
User-friendly interface provides fast and logical data entry for application
Transparent credit application process and feedback report with suggestion in case of rejected application

Automated credit application process and reports save time and effort
Increase the chance of making profitable credit decisions and reduce risks
Multiple customization options, access to extensive database and versatile comparison tools

Receive standardized credit reports on case companies
Increase transparency between investor and broker
Loans granted by using Valuatum’s software are more accurately rated
Compare how Valuatum’s model outperforms the prevailing way to do credit risk analysis
PREVAILING WAY
PROCESS
APPROACH
- Use of inaccurate and outdated models and methods where bankruptcy risk is often estimated just by looking at a few financial ratios
- Does not take into account the individual characteristics of case companies
RESULT
- Prevailing way places all companies into same mold, failing to estimate bankruptcy risk accurately in cases where individual approach is needed
VALUATUM WAY
PROCESS
- Most of the time consuming tasks are automated
APPROACH
- Based on advanced models and methods where bankruptcy risk is estimated by dynamically weighting financial ratios
- Takes into account the unique nature of each case company
RESULT
- Valuatum’s way chooses the most suitable financial ratios and adjusts weights to fit each scenario
- Bankruptcy risk is estimated accurately for each individual case, leading to efficient and accurate credit risk analysis
Real-life examples of how traditional credit rating estimation methods might fail
Examples below will provide some insight on cases that highlight the superiority of modern machine-learning algorithms over more traditional models that use fixed weights. In all cases fixed-weight models might easily lead into costly mistakes, while dynamically weighting the most important variables in each case will help to concentrate on what really matters.
EXAMPLE 1
COMPANY PROFILE
- Company A’s business is relatively stable. It has a high profitability with a good financial standing. Thus they don’t need to maintain good liquidity because they can easily get credit from the banks if needed.
CREDIT RISK ESTIMATION
- Due to fixed weights, prevailing model(s) overemphasize the impact of liquidity. This makes Company A’s estimated bankruptcy risk to be higher than it really is, which also makes its credit rating worse.
- Machine-learning models will correctly put less emphasis on liquidity and concentrate on other figures instead.
RESULT
- Traditional models might cause the lender to miss a good low-risk investment opportunity due to too high weighting on liquidity where it does not matter much. Moreover, Company A’s interest rate is higher than it should be.
- Machine-learning models recognize that liquidity is less important with companies like Company A and therefore assign a correct credit rating and allow lender to capitalize on a low-risk opportunity. Company A is offered the interest rate it deserves.
EXAMPLE 2
COMPANY PROFILE
- Company B has poor profitability and it is making heavy losses. It has a weak financial standing with high indebtedness. Thus it doesn’t have buffers to face any setbacks.
CREDIT RISK ESTIMATION
- With these type of cases all comes down to liquidity and models with fixed weights often under-estimate its impact. This makes Company B seem more creditworthy than it really is.
- Machine-learning models will understand that the importance of liquidity increases in cases like Company B and correctly put more emphasis on it and less emphasis on other figures.
RESULT
- Using traditional models, lender might make too risky of an investment based on inaccurate credit rating caused by poor liquidity.
- Using machine-learning models, lender would be able to correctly evaluate the riskiness of the investment and assign an appropriate credit rating and interest rate.
EXAMPLE 3
COMPANY PROFILE
- Company C is highly profitable and has relatively low indebtedness, making C look like a solid low-risk company. However, upon closer inspection it is noticed that the company has high sales receivables turnover time and balance sheet consists of almost exclusively sales receivables
CREDIT RISK ESTIMATION
- Models with fixed weights assign a good credit rating due to high profitability and low indebtedness, paying little attention to potential underlying problems.
- Machine-learning models are able to recognize that despite seemingly good financial standing, the high receivables turnover time might indicate that the company is actually in a bad situation financially.
RESULT
- Using traditional models, lender might make too risky of an investment based on inaccurate credit rating.
- Machine-learning models are able to flag the company and the lender knows to look into the details and check the actual financial state of the company, potentially allowing them to dodge a high-risk investment.
Key features of Valuatum Credit Risk Platform
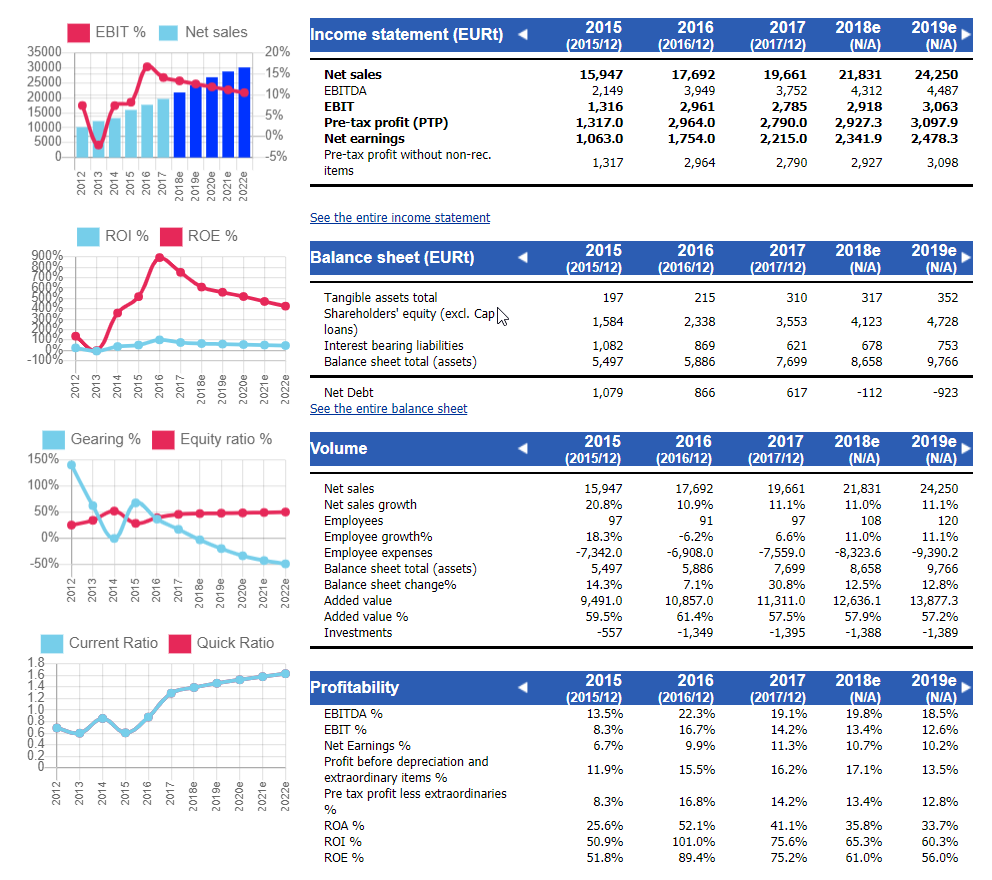
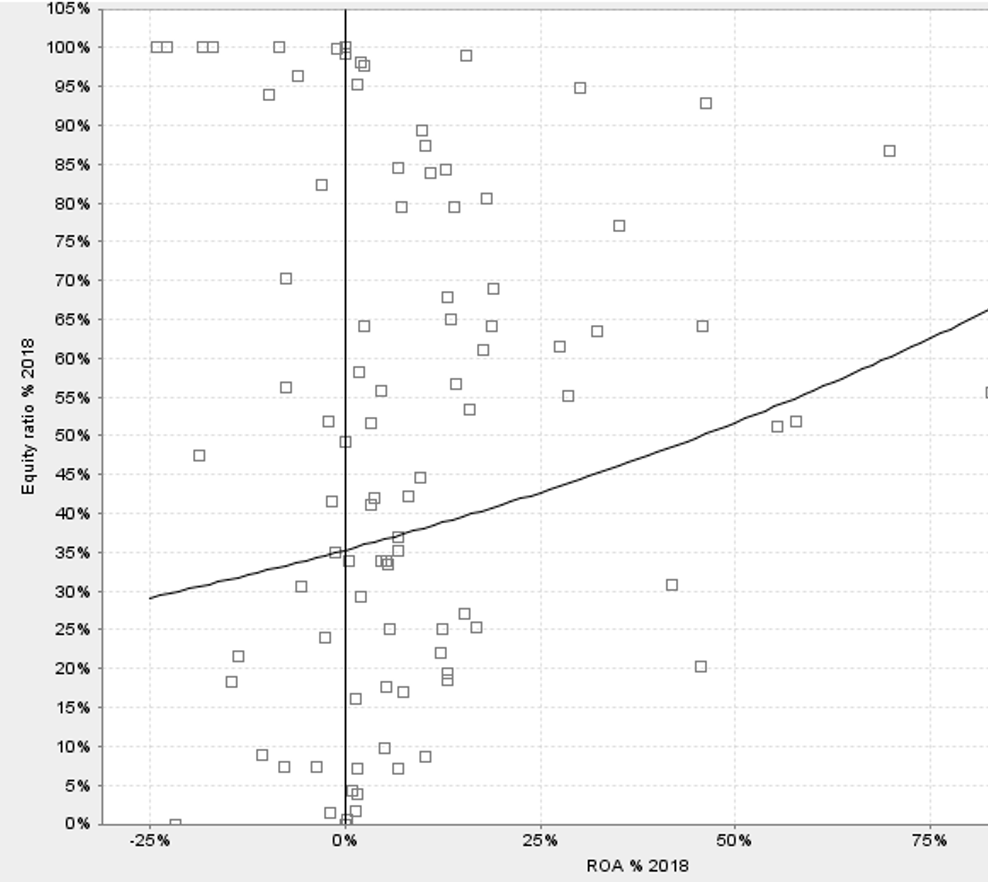
Comparison tools
Peer group analysis
Versatile selections
Hundreds of variables
Lists and scatter plots
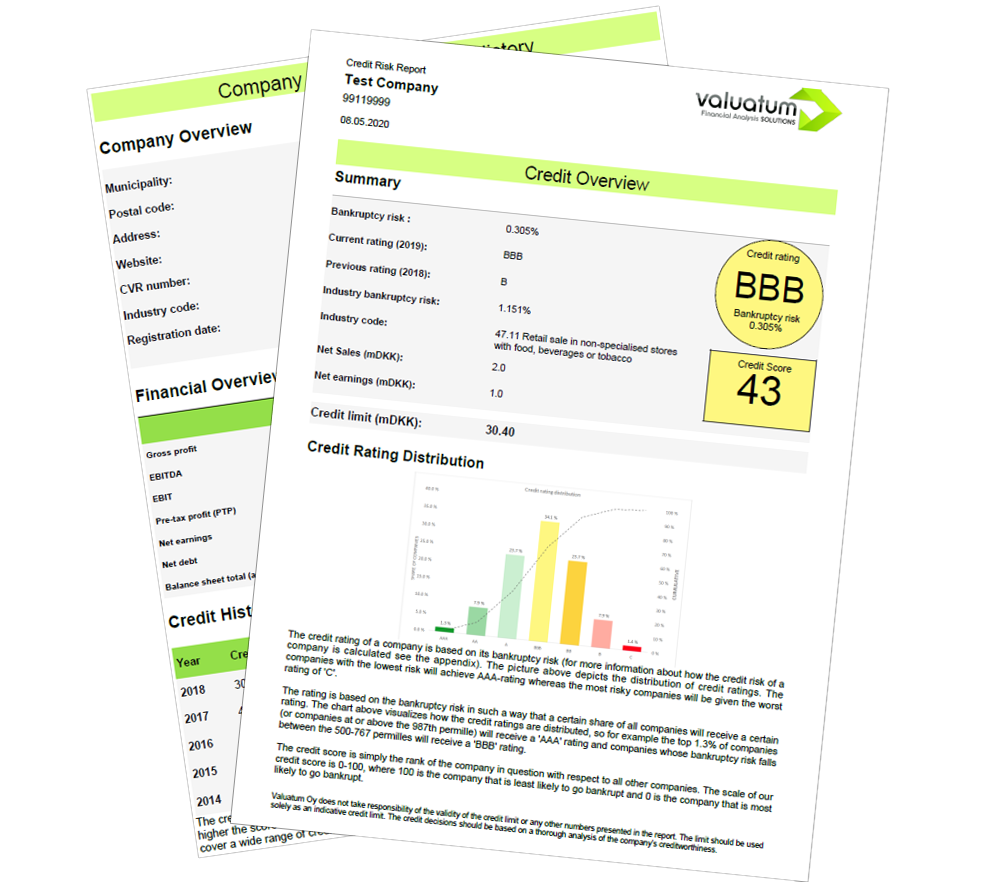
Automatic reports
Pre-filled tables and graphs
Customizable layouts
PDF and Word formats
One-click printing
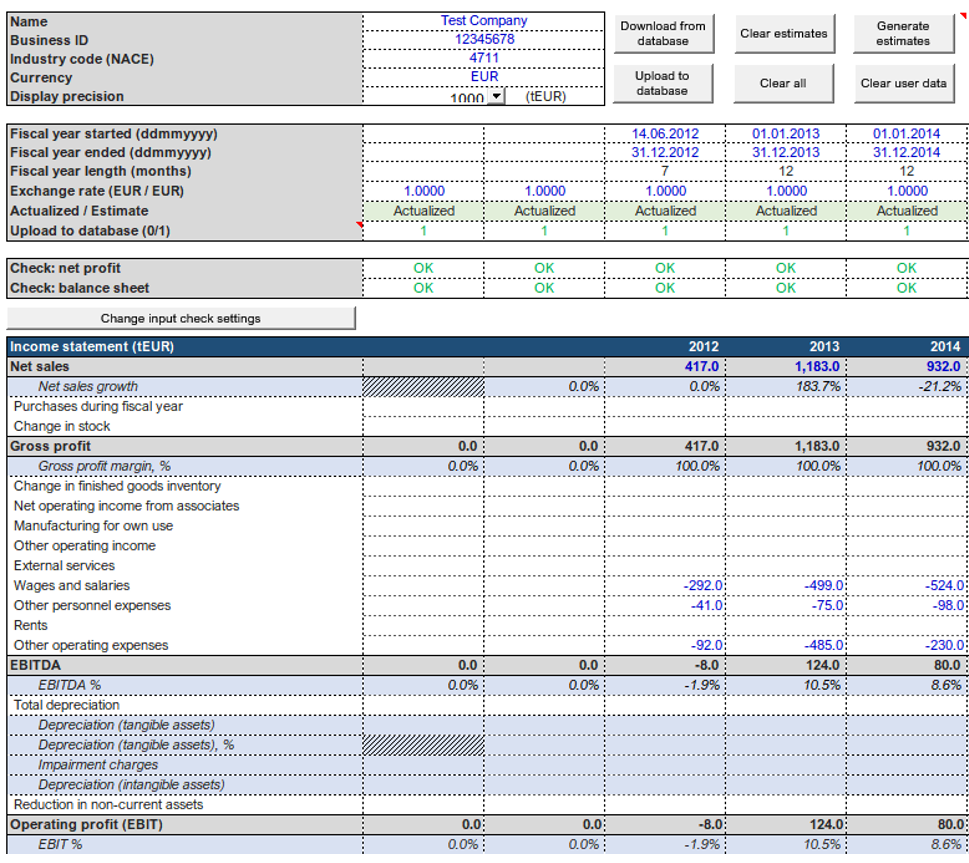
Excel models
Edit financial data effortlessly
User friendly interface
Automatic features
Support for custom sheets